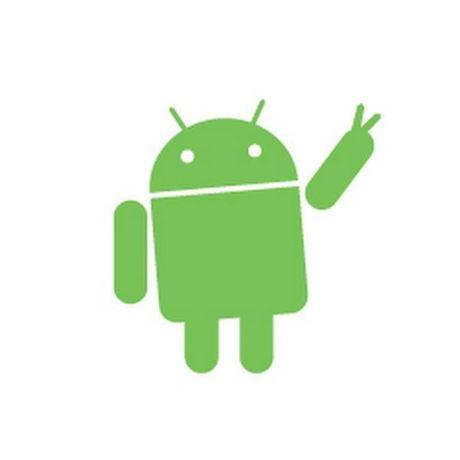
- Projects 18
- Followers 12
Experiment 1: Brain Tumor Recognition ...learn more
A brain tumor is a growth of abnormal cells in the tissues of the brain. Brain tumors can be benign, with no cancer cells, or malignant, with cancer cells that grow quickly. Some are primary brain tumors, which start in the brain. Others are metastatic, and they start somewhere else in the body and move to the brain. Brain tumors are the second most dangerous cancer in children, yet knowledge about this cancer is insufficient. Although in early childhood this accounts for about as much as all the other solid tumors in the body combined, the general public and clinicians tend to know much less about brain cancer in children than leukaemia. Despite some progress being made over the last 2 decades, overall patient outcomes remain poor when compared with other childhood cancers.
According to Department of Neurosurgery, University of Cape Town‘s Prof Anthony Figaji, “There remains much uncertainty about the nature of various brain tumors and their prognosis. In particular, virtually nothing is known about children with brain tumors in a South African setting and no current research projects address this. Fortunately, there is a growing awareness of the lack of research in non-communicable diseases in South Africa, which is timely because there has been
exciting progress in tumor biology research elsewhere, especially in the last 5 years, that promises to open new avenues
to better classify and treat various brain tumors. These are revealing important genetic origins of these cancers and may
lead to targeted treatment for individual subgroups of brain tumors. These subclassifications likely will be a requirement in
the future for international clinical trials.”
Glioma are the most common family of brain tumors, with a subset of glioma known as glioblastoma forming the most
common and some of the highest-mortality and economically costly forms of brain cancer. Patients are diagnosed based on
manual segmentation and analysis of multimodal MRI scans, but due to the labor-intensive nature of the manual
segmentation process and mistakes or disagreement between manual segmentations, there exists a need for a fast and robust automated segmentation algorithm. Convolutional neural networks (CNNs) have been shown to be
extremely effective for a variety of visual recognition and semantic segmentation tasks.
Here we present TMIP_BrainDetection model to classify brain tumors from a dataset of 32 patients, tumour features such as size, enhancement quality, necrosis proportion, etc. were extracted by radiologists. Diagnosis was also established for these patients. Based on this information we were able to create an optimized model to classify tumors with a 90% cross-validated accuracy. We used preprocessing techniques to clean data, model selection (Logistic Regression, K Nearest Neighbor, Niave Bayes and CNN) and glioma segmentation. In this project, we built a model to classify brain
tumors into three types based on MRI scans: Astrocytoma, Oligodendroglioma or Glioblastoma.
Goal and success criteria
Our goal is to build a classifier that performs better than the baseline (80% accuracy for the 32 patient dataset and 70% for the whole dataset). With the aim to predict probabilities of each tumor type in order to assist radiologists in their diagnosis. Thus, we shall compare several models (such as Logistic Regression, Naive Bayes, Random Forest, KNN and CNN) based on their accuracy, confusion matrices and ROC curves and select the most optimized one.