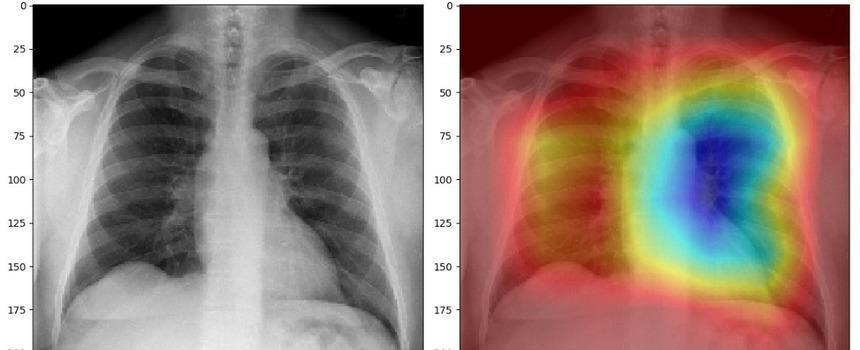
TREATISE OF MEDICAL IMAGE PROCESSING: COVID-19 RECOGNITION
A convolutional neural network-based method for recognition of COVID-19 in Chest X-Ray and Computed Tomography (CT) radiographs, and a method for medical image processing of large datasets related to COVID-19. The medical image processing method comprises:: 1. Data Collection, 2. Data Processing , and 3. Training a convolutional neural network. Using the Intel oneAPI DevCloud and Intel® AI Analytics Toolkit, we are able to quickly get started and focus on the task of building and training the intelligent COVID-19 prediction model using Intel optimized Tensorflow for CPUs available in oneAPI DevCloud.
INTRODUCTION
On Dec 31st the World Health Organization was made aware of an illness showing similarities to respiratory pneumonia with symptoms that include a fever, cough and shortness of breath. The origin of this virus is believed to be in Wuhan City, the Hubei Province of China and is officially known as COVID-19. The virus belongs to a genome (the genetic material of an organism), that includes SARS Severe Acute Respiratory Syndrome and MERS Middle East Respiratory Syndrome.
Given the almost exponential rise of infection rates world-wide, early detection of the disease's presence is essential not only to ensure prompt treatment but also to help with the management and control of infection rates in the public domain. The high infection rates and the shortage of COVID-19 test kits available, increases the necessity of the implementation of an automatic recognition system as a quick alternative to curb the infection rates.
We thus propose the use of an AI based analytics system for chest scans to detect COVID-19 pathogens under the project Treatise of Medical Image Processing (TMIP) v0.2.0. Using an AI based analytics system for chest scans methodologies and implementations portrays the project’s potential to combat the increasing burden and diagnostic downtime heavily dependent on a limited number of well-trained radiologists and medical experts, who must review and prioritize an increasing number of patient chest scans. The system is designed to process large numbers of chest scans per day. As a result, the system will help predict which patients are most likely to need a ventilator or medication, and which can be sent home for self-quarantine. Thus, the solution will contribute to the fight against COVID-19 pandemic in three ways: identification, monitoring and predicting patient status.
The solution is designed to employ Intel optimized machine learning hardware and software technologies to train, test, and operationalize a model to help detect COVID-19 and 14 other thoracic diseases using chest scan. Early diagnosis and treatment of COVID-19 and other lung diseases can be challenging, especially in geographical locations with limited access to trained radiologists. Using the Intel® AI Analytics Toolkit and other tools, services and infrastructure provided by the Intel oneAPI DevCloud our data scientists could quickly iterate and train deep learning models which have the potential, following further development and testing, to classify diseases from chest scans.
In this project, we use the following resources:
- Dataset: For confirmed COVID-19 cases we collect data from open source chest x-ray dataset (COVID-19 Chest X Ray-Dataset).We also used the National Institutes of Health Clinical Center public Chest X-Ray dataset RSNA ( RSNA Pneumonia Detection Challenge on Kaggle dataset.)
- Machine Learning Frameworks: To build COVID-19 Recognition Deep Neural Networks based on input images from X-Ray scans we employed Intel® Optimized Tensorflow. Base architectures we experimented with the state-of-the-art DenseNet , ResNet, and ChexNext for image classification. All of the models used are open-source deep learning algorithms with implementations available in Keras (using Intel® Optimized TensorFlow as a back-end).
- Hardware Accelerators: To build a COVID-19 Recognition model we requested access to the Intel oneAPI DevCloud. We thus trained the model with full access to the latest Intel CPUs, GPUs, and FPGAs, Intel oneAPI Toolkits, and the new programming language, Data Parallel C (DPC). This helped accelerate our training time from 48 hours using our developer machines (i.e, laptop) to 6 hours using oneAPI DevCloud.
Data Preprocessing
The use of X-Ray is inexpensive and quick to perform; therefore, they are more accessible to healthcare providers working in smaller and/or remote regions. Any insights that may be derived as a result of explainability algorithms applied to a successful model will be invaluable to the global effort of identifying and treating cases of COVID-19. We used COVID-19 Chest X Ray dataset, one of the largest public repositories of COVID-19 radiographs, containing about 400 frontal-view chest radiographs of 549 unique patients. Each image in the dataset was labelled by radiologists from different hospitals where patients infected with COVID-19 were diagnosed. Furthermore, we used the RSNA Pneumonia Detection Challenge dataset from Kaggle as the non-COVID-19 dataset. Implementing accelerated data science and analytics pipelines, preprocessing through machine learning, and scale-out efficiently using the high-performing oneAPI Data Analytics Library, part of the foundational Intel oneAPI Base Toolkit. The library’s set of high-speed algorithms (such as analysis functions, math functions, and training and prediction functions) enable applications to analyze large data sets with available compute resources and make better predictions faster.
Working on the COVID-19 detection problem, we also experimented with various hyper parameters to improve the performance of the deep learning models, focusing on the lungs. Specifically, we explored how to detect the lung location in the chest x-ray, and crop out irrelevant areas by using Intel optimized Tensorflow framework. These chest X-Ray scans are then provided as inputs to DenseNet. We have also published the code on GitHub, this solution is written using the High-Performance Intel distribution of Python, one the features of the Intel AI Analytics Toolkit.
Machine Learning
We propose the use of Deep Neural Networks. As an initial experiment the DenseNet architecture was used as a baseline where transfer learning is employed to detect pneumonia. For training we employed the Intel-optimized TensorFlow framework from Intel AI Analytics Toolkit that has been optimized using Intel(R) Deep Neural Network Library (Intel(R) DNNL) primitives. Deep learning frameworks provide a high-level programming language to architect, train, and validate deep neural networks. Model training process consists of 2 consecutive stages to account for the partially incorrect labels in the COVID-19 dataset. First, an ensemble of networks is trained on the training set to predict the probability that each of the 14 pathologies is present in the image. The predictions of this ensemble are used to relabel the training and tuning sets. A new ensemble of networks are finally trained on this relabeled training set. Without any additional supervision, the model produces heat maps that identify locations in the chest radiograph that classify COVID-19 among other pathologies
Performance
Following the machine learning best practice, we use the AUROC score to measure the performance for the classification of COVID-19 by selecting the model with the lowest validation loss.
The ROC curve (receiver operating characteristic curve) shown in figure 4, is a graph showing the performance of a classification model at all classification thresholds. An ROC curve plots TPR vs. FPR at different classification thresholds. Lowering the classification threshold classifies more items as positive, thus increasing both False Positives and True Positives.
To compute the points in the ROC curve, we evaluate the AUC (Area under the ROC Curve).That is, AUC measures the entire two-dimensional area underneath the entire ROC curve from (0,0) to (1,1). Thus, the AUC provides an aggregate measure of performance across all possible classification thresholds.
The result we obtain from the model over a period of 70 epochs is plotted in Figure 5. The average AUROC across all the epochs is 0.9743. That is, our model's predictions are 97.43% correct on average across all classification thresholds.
We followed the science of data analytics general practices to evaluate the models performance using AUC. Thus, AUC is desirable for two main reasons:
- AUC is scale-invariant, thus it measures how well predictions are ranked, rather than their absolute values
- Classification-threshold-invariant, thus measures the quality of the model's predictions irrespective of what classification threshold is chosen
Explanation
-
Locating COVID-19 Using Class Activation Mapping (CAM ): We use CAM, a technique for producing "visual explanations" for decisions from a large class of CNN-based models, making them more transparent. CAM images empower data scientists to visualize the gradient of the label in the final convolutional layer to produce a heatmap depicting regions of the image that were highly important during prediction.
-
Locating COVID-19 Using Local Interpretable Model-Agnostic Explanations (LIME). For higher level interpretability, understanding and explaining our model predictions we employ LIME.
Conclusion
The experimental findings showed how we used Intel® AI Analytics Toolkit and Intel oneAPI DevCloud to train, test, and operationalize a model to help detect COVID-19 and other thoracic diseases using chest x-ray images. Early diagnosis and treatment of pneumonia and other lung diseases can be challenging, especially in African countries with limited access to trained radiologists and medical staff. Using the tools, services and infrastructure provided by Intel, data scientists can quickly iterate and train deep learning models which have the potential, following further development and testing, to classify diseases from chest x-ray images. This model is a prototype system and not for medical use and does not offer a diagnosis.
Special Thanks to the following contributors:
Tibrewala, Sujata (Intel)
Venkatesh, Preethi (Intel)
Oberman, Rachel (Intel)
Satish, Saumya (Intel)
Kay-lee Abrahams (University of Cape Town)
Shahram Rezasade (Accrad Technologies)
Related Links
- Source Code: https://github.com/TebogoNakampe/TMIP-2019-nCoV-Recognition
- LIME: https://arxiv.org/pdf/1602.04938.pdf
- CAM: https://arxiv.org/abs/1610.02391
- MS Azure: https://docs.microsoft.com/en-us/archive/blogs/machinelearning/using-microsoft-ai-to-build-a-lung-disease-prediction-model-using-chest-x-ray-images
- Inte AI Kit Code: https://github.com/intel/AiKit-code-samples
- Intel AI Kit Home: https://software.intel.com/content/www/us/en/develop/tools/oneapi/ai-analytics-toolkit.html
- Google Dev’s: https://developers.google.com/machine-learning/crash-course/classification/roc-and-auc